随笔:一些DL编程中的小"寄"巧
近期一些DL编程中的小"寄"巧
一. python numpy -- tofile,fromfile,save,load
参考资料: https://blog.csdn.net/weixin_39087379/article/details/118048780
笔记:
tofile
和fromfile
是一对,二进制读写进文件。由于不保存数组形状和元素数据格式等信息,因此一定要设置正确的dtype
参数,并且reshape
正确的形状。
举例,SemanticKitti的语义标签数据读取np.fromfile(path, dtype=np.uint32)
。当时犯了2个错:
- 首先使用
np.load
读取的.label
格式文件,导致读入的Ground-Truth标签一直有误,且数量和点云数量对不上。 - 换成
np.fromfile
后,dtype
忘记设置导致格式不对。除此之外, NuScenes的label也需要np.fromfile
打开.一般来说,tofile
和fromfile
处理的是.bin
后缀的文件,load
和save
处理的时.npy
后缀的文件。
1 |
|
save
和load
是一对,是Numpy
专用的二进制格式保存数据,可以自动处理元素类型和形状信息。 之前犯过的错误有以下几种:
np.load
下意识的设置dtype
反而报错- 错把普通二进制文件用
np.load
打开导致数据错误
1 |
|
此外numpy.savez_compressed
可以把多个array存到一个文件中并压缩.如下面例子:
1 |
|
savetxt()
和loadtxt()
,读写1维和2维数组的文本文件;也可以用它们读写CSV格式的文本文件
例子:
1 |
|
二. 关于Pytorch分布式训练DistributedDataParallel中的一些坑
说明:
博主目前还在用着落后的python -m torch.distributed.launch -nproc_per_node=8 XXX.py
的方式启动,
还没学pytorch官方推荐的torchrun
此外不太理解DDP的小伙伴可以先读一下博客理解一下
如果你的神经网络中有batchnorm层, 一定要在模型从cpu移到gpu前(
my_model.cuda()
)加上这一句,让batchnorm参数在各个节点之间建立同步关系.Currently SyncBatchNorm only supports DistributedDataParallel with single GPU per process. Use torch.nn.SyncBatchNorm.convert_sync_batchnorm() to convert BatchNorm layer to SyncBatchNorm before wrapping Network with DDP.
1
2
3
4
5
6
7# DDP的sync_bn,让多卡训练的bn范围正常
if args.local_rank != -1:
my_model = torch.nn.SyncBatchNorm.convert_sync_batchnorm(my_model)
my_model.cuda()
if args.local_rank != -1:
my_model = torch.nn.parallel.DistributedDataParallel(my_model, device_ids=[args.local_rank],
output_device=args.local_rank,)参考资料: pytorch官方文档自己去搜
Sampler | Dataloader | |
---|---|---|
单卡 | torch.utils.data.sampler.Sampler | torch.utils.data.DataLoader |
Distributed Parallel | torch.utils.data.distributed.DistributedSampler(train_dataset) |
torch.utils.data.DataLoader |
其实Pytorch是通过继承Sampler的不同子类,来实现对DIstributedParallel的支持. Dataloader提供的接口时一样的(做个比喻: 仓库还是同样的仓库, 只不过从原来的一个worker干活变成多个worker分头干活, 所需要的调度器sampler不同).
这里就有一个坑: train_sampler.set_epoch(epoch)
这一项一定要在dataloader的实例生成迭代器(iterator)之前设置好,否则无法在多个节点上通过sampler实现shuffle数据的功能
(前提是torch.utils.data.DataLoader(my_dataset, sampler=train_sampler)
设置好的基础上,因为sampler
和shuffle=True
不能同时设置).
例如
1 |
|
补充一个小福利:https://github.com/huggingface/transformers
中好像有SequentialDistributedSampler
的实现,
好像很多人都在使用.
在分布式并行时,这个类用于数据集的Eval和Test顺序读取比较好用.
Demo代码--快来戳我┗|`O′|┛ 嗷~~
1 |
|
1 |
|
分布式中节点间传递消息: 除了通信的API之外(
reduce
,gather
,all-gather
等), 有一种最简单最傻的方式, 按照某个格式保存到本地文件,然后只让0号卡负责读取,其他的进程torch.distributed.barrier()
. 这种对于大量数据的传递比较好用. 应用实例: 比如有一个自己手工作坊制造的类, 在单卡上很好用,但是在多卡上你发现改写成分布式的太麻烦了(比如涉及到读写冲突,进程通信同步等问题)烦死了, 你不想浪费宝贵的科研时间单纯改造这个类, 不妨试试上面的傻瓜方法.分布式训练出来的权重再次load时候
1 |
|
--- (2024.07更新) ---
- 在使用多卡推理的时候,建议每个DDP进程把自己负责的每个图片文件的推理结果都同步过来,类似
1 |
|
来做多进程结果手机。这样多卡推理时metric计算误差最小,基本和单卡推理结果一模一样。不太建议比如每张卡先算个平均的分指标/求和算个总指标,然后只同步一个总的数字,然后再8个节点上算平均,这样误差大(甚至波动1个点)。
- 注意tensorboardX使用summary
writer记录日志时,最好只用一个进程(比如rank0)来创建,或者每个进程日志创建地址名字结尾用进程名字作为隔离
(f"XXX_tb_process_{rank}")
,不然一直报错"File Exists"错误。 - 通过如下方式,只对rank0进程上使用tqdm避免显示混乱。
_data_loader = tqdm(_data_loader, ascii=True) if self.rank==0 else _data_loader
- DDP多卡从obs远程下载/读取文件到云节点的Docker时,一定注意避免多个进程读/写同一个文件导致一致性冲突。比如如果在
__init__
阶段需要copy同一个文件到本地(8个进程都需要),不如提前下载好到本地,然后执行代码;或者8个进程下载8次,每次文件名后缀用f"_process_{rank}"
区分,,如果在__get_item_
阶段读取文件,因为数据集会被8个进程完整分成8份互不相交的文件,不应该有读写冲突。所以关注不同文件名下的文件有没有重名现象,避免重名造成一些隐性的error。 - 注意算推理时间的时候,还是尽可能把可视化和文件读写关闭一下。因为关闭可视化和文件读写,有可能会省去网络模型的某些操作,算FPS和Latency时能更快。
三. Pytorch 中节约时间开销的一些方法(持续汇总)
(最新,亲测非常好用)
torch.set_float32_matmul_precision("highest|high|medium")
pytorch参考doc: 直接搜
set_float32_matmul_precision
相关博客解释:https://blog.csdn.net/sikh_0529/article/details/131243173 【使用混合精度技术加速大型语言模型】总体来说,就是Float32充分利用NVIDIA显卡中的TensorCore模块,原先做Tensor乘法完全用Float32的数据格式做,速度自然慢
性能对比:转自上述博客,开启high模式混合精度矩阵乘法,速度快几倍且精度能略微上涨。博客解释原因是1. 深度网络对精度的适当下降,如从FL32变为BF32格式,不会有收敛性上的太大损失;2.精度适当的截取下降,配合上混合精度,可以给网络带来更多噪声,有利于防止过拟合,增强泛化能力。目前在自己代码上亲测效果,4090能加速5-6倍的训练速度,精度目前还在调bug中。
支持条件:
torch.cuda.is_bf16_supported()
总原则:CPU 把算子和任务下发到CUDA上。尽可能不要让CUDA等CPU,CPU一次性把没有数据依赖的算子下发完毕,然后让cuda去算。(下发的斜线越倾斜越好,cuda stream越满越好)
在前向传播中,尽量少出现.to(device), .cpu()等搬运数据的操作;可以用torch.arrange(, device="cuda")直接把数据放在cuda上,或torch.oneslike(, device="cuda")
Avoid unnecessary CPU-GPU synchronization
Avoid unnecessary synchronizations, to let the CPU run ahead of the accelerator as much as possible to make sure that the accelerator work queue contains many operations.
When possible, avoid operations which require synchronizations, for example:
print(cuda_tensor)
cuda_tensor.item()
- memory copies:
tensor.cuda()
,cuda_tensor.cpu()
and equivalenttensor.to(device)
calls cuda_tensor.nonzero()
- python control flow which depends on results of operations performed
on cuda tensors e.g.
if (cuda_tensor != 0).all()
- 更多参见: https://pytorch.org/tutorials/recipes/recipes/tuning_guide.html
有人建议把GPU显存利用的满一些(batchsize 开大一点). 但是通过实验好像发现,对于20系和30系的N卡, 有效显存大概6G~8G左右,也就是CUDA数量一次能够处理的最大显存容量. 如果超过这个容量的数据还是会放在显存里. 通过自己的代码多次实验发现,基本显存占用>8G后, batch-size开的再大总时间也不会减少了.
图像用lmdb(不过不一定必须,当CPU利用率还有充足空间,硬盘不是太慢如本来就是SSD时,这个不一定是瓶颈)
自己profile, 找到代码的加速瓶颈
使用pytorch.amp混合精度加速(开O1)
AutoDL 显卡租用平台的官方文档中:https://www.autodl.com/docs/perf/ 总结的非常好!此外,AutoDl还推荐了Pytorch官方的小技巧总结: https://pytorch.org/tutorials/recipes/recipes/tuning_guide.html.
四. Pytoch 中节约显存开销的一些方法(持续汇总)
torch.cuda.empty_cache()
,每跑完一个Epoch用一下,防止显存占用一直增长 (好像在OpenMMLab家族系列的框架中包含了这一点)稀疏化的tensor表达会省很多显存,尤其是在计算
CE_loss
的时候,稀疏化的CE_loss
会给反向传播节省很多显存.太大的变量不要一直等着自动回收,可以手工定期delete一下
torch.load(XXX.pt, map_location="cpu")
, 可以避免在开多卡时, 某张卡的显存占用明显比其他卡多2~3个G导致整个程序OOM.
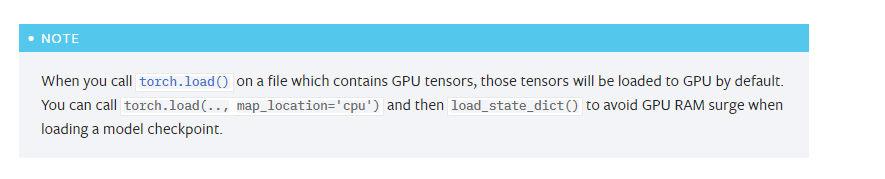
发现的一个现象:pytorch中的dataset的num_workers,如果开少了可能CPU难以微保GPU,导致GPU空等CPU利用率不高;但是如果num_worker开的过高,比如一口气开到10以上(我有一次开到过32),会出现:
- CPU 核被占的非常多,如果这台机器就你自己用还好,如果多人用,别人的程序也会被你连累了,变得特别慢;
- 内存开销会陡然增加很多,会导致容易内存OOM,程序训练了几十个Epoch后不知道啥时候突然寄了,还没有报错原因,或者报错原因是signal 9 killed,因为超过内存被系统kill调了。如果是在物理机上,由于有硬盘可以作为虚拟内存的机制,或许能缓冲一下不会直接挂掉; 但如果是在容器里环境基本立马被干掉。
- pytorch中num_workers开多了会放大内存泄露的风险。某一次实验观察发现:某多卡DDP的训练程序,开了num_workers=4,看内存占用曲线图有少量的内存泄漏,但是训练300多个Epoch一共泄漏不到几十G;但是一旦开到num_workers=32,瞬间十几个Epoch后就泄露了100多G。而且这种num_workers的现象在mindspore框架最初1.1~1.5版本中也出现过内存泄漏现象。估计这都是深度学习框架的通病吧,不能开太大了。。。
(4)补充:在Docker中,如果单卡训练且num_workers开的过大,可能会在遍历dataloader取数据的过程中,超过Docker默认的最大打开文件数量,会报错“[OSError 26]: Open too many files.” 的错误而强制退出。
五. Pytorch 关于Tensor下标操作的一些技巧
常用的一些函数如:
torch.all(), torch.nonzero(), torch.where(), torch.masked_fill(), torch.masked_select(), torch.scatter_select()
等等,PyTorch提供了非常丰富的下标操作函数,尽可能让我们避免在__forward__()
函数中使用for循环,因为for循环需要CPU串行参与计算,无法完全实现在GPU上并行化的计算。
1 |
|
此外还要区分一下torch.stack()和torch.concat()的区别:
torch.stack(): Concatenates a sequence of tensors along a new dimension. All tensors need to be of the same size. 所有尺寸必须相同,是要新建一个dim维度然后再新维度上把多个尺寸相同的拼起来。
torch.cat(): concatenates the given sequence along an existing dimension. 已有维度上变得更宽。除了dim选择的维度大小可以不一样,其他维度尺寸必须相同。
1 |
|
高维空间不容易想象,建议最好用简单的二维情况处理。如果需要高维,建议用小数据量多做做实验。比如点云Voxel中常见(BatchSize, Num_Cameras, Channel, X_axis, Y_axis, Z_axis) 形状的Tensor,你要凭空用脑袋去想对某个dim进行stack或cat等操作,脑子都要炸了。建议用几个简单样例跑一跑,纸笔画画图,具体情况具体分析。